일 | 월 | 화 | 수 | 목 | 금 | 토 |
---|---|---|---|---|---|---|
1 | 2 | 3 | 4 | 5 | ||
6 | 7 | 8 | 9 | 10 | 11 | 12 |
13 | 14 | 15 | 16 | 17 | 18 | 19 |
20 | 21 | 22 | 23 | 24 | 25 | 26 |
27 | 28 | 29 | 30 |
- XGBoost
- 부트 스트래핑
- splitlines
- 데이터 증식
- lightgbm
- 캐글 산탄데르 고객 만족 예측
- 그로스 해킹
- 그룹 연산
- WITH CUBE
- 인프런
- 데이터 핸들링
- 스태킹 앙상블
- 컨브넷
- Growth hacking
- DENSE_RANK()
- 3기가 마지막이라니..!
- python
- tableau
- 그로스 마케팅
- 분석 패널
- 캐글 신용카드 사기 검출
- pmdarima
- 리프 중심 트리 분할
- 로그 변환
- WITH ROLLUP
- sql
- ARIMA
- 마케팅 보다는 취준 강연 같다(?)
- 데이터 정합성
- ImageDateGenerator
- Today
- Total
LITTLE BY LITTLE
[2] 캐글 RSNA 2022 Cervical Spine Fracture Detection - Literature Review 선행 연구 본문
[2] 캐글 RSNA 2022 Cervical Spine Fracture Detection - Literature Review 선행 연구
위나 2022. 10. 14. 22:19** Cervical Spine Fracture (경추 골절)
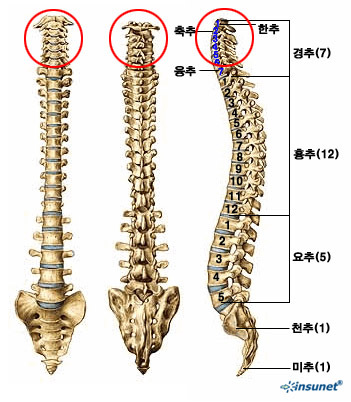
1. 정의 : 손상을 입을 때 가해지는 힘의 작용에 의해 경추에 다양한 형태의골절이 발생한 것
2. 증상 : 목뼈의 몸체 일부가 떨어져 나가는 것을 눈물방울 골절(눈물방울 형태로 떨어져 나갔기 때문에 이런 이름을 얻음)이라 하고, 뒤로 나온 뼈가 부러지는 것을 극돌기골절, 양측횡돌기가 부러지는 것을 횡돌기 골절이라 한다
3. 원인 : 교통사고와 관련된 외상이 가장 많고, 추락사고, 다이빙 사고, 격렬한 운동경기 중의 사고에서 발생하며 목이 휘청거리는 등의 간접적인 힘으로 생길 수 있다
4. 치료 : 목뼈가 흔들리는 불안정성이 없고 신경손상이 없으면 경추 보조기를 착용하고 투약하며 안정을 취하면 호전된다. 척추에 불안정이 있거나 척추가 어긋난 경우에는 골 견인 후 골 유합술을 실시한다. 외상성 경추간판 탈출증이 있어 신경을 압박하거나 부러진 뼈 조각이나 어긋난 척추에 의해 신경이 압박되면 감압술을 하여 신경이 압박되는 것을 제거하고 척추를 고정한다
5. 합병증 : 신경손상이 있으면 사지마비, 호흡마비, 방광기능의 이상이 오고 그로 인해 장기간 또는 평생 누워서 지내게 되고, 욕창, 폐렴, 요로계 합병증(방광염, 결석증), 정맥혈전증, 폐전색증, 골조송증 및 자발성 골절 등이 생길 수도 있다.
보고서의 구성
1. 서론: 배경 및 문제 설명
2. 선행연구: 문제와 관련된 기존 연구나 모델 리뷰 Method: Explain your statistical models for the problem
3. 제안방법: 제안하는 방법 및 모델(기법)
4. 실험
4.1 데이터 및 실험계획: 모델 학습에 사용할 데이터 설명; 모델 학습 계획; 평가지표
4.2 실험결과: 제안하는 모델의 성능 및 다른 모델과의 지표 평가(제안서 발표 시는 생략)
5. 결론: 프로젝트의 요약 및 정리; 결과로부터의 결론; 제안한 모델의 장단점; 향후 연구 논의 등(제안서 발표 시는 생략)
2. 선행연구: 문제와 관련된 기존 연구나 모델 리뷰 Method: Explain your statistical models for the problem
: " 크게 세가지 선행연구를 참고하였다. 첫 번째는 현재 척추 이미지 분석에 쓰이고있는 CNN의 성능을 평가한 논문으로, 현재 척추 CT 스캔에 쓰이고 있는 CNN은 속도가 빠르고 정확도가 높아 이전에 X-RAY보다 좋은 성능을 보이고 있긴 하나, 여전히 FP,즉 골절이 아닌데 골절이라고 예측하는 경우가 많다는 한계점이 존재한다고 지적하였다. 그 예시로 척추 앞 부종, 디스크 확장과 같은 2차 징후, 선천적 이상, 그리고 수술 이후 변화와 골절을 구분하지 못하여 골절이 있는데에도 불구하고 놓치는 경우가 많이 있었다. 두 번째로는 현재까지 척추 이미지 분석에 쓰이고있는 84가지의 딥러닝 네트워크를 리뷰한 글을 참고하였다. 네트워크의 35.4%는 classificaion, 30.4%는 segmentation이 차지하고 있었으며, CNN과 U-Net이 압도적으로 많았고, 분류에서는 FCN이, 분할에서는 LSTM이 상대적으로 많이 쓰였다. 마지막으로 갈비뼈 골절을 진단하기 위해 고안된 두 단계 모델을 제안한 논문을 참고하였다. 갈비뼈 진단을 위해 만들어진 FracNet은 3D U-Net에 기반하여 segmentation하고, ResNet을 분류모델로 사용하고 있었으며, 그 외에도 CNN이 segmentation 과정 없이도 99.1%의 높은 정확도를 보이고 있었다. 하지만, 기존의 네트워크는 대부분 2 dimensional basis라는 한계점이 있었기 때문에, 본 연구에서는 기존의 U-Net을 수정한 nnUnet과 DenseNet으로 구성된 알고리즘을 제안하였다. 활성화 함수 ReLu를 leaky Relu로, Batch Norm을 Instance Norm으로 대체하였으며, segmentation을 하고 분류하기 이전에, 한번 더 False Negative를 제외시키는 과정을 거쳤다는 것이 특이점이다. "
선행 연구 요약
1. CT Cervical Spine Fracture Detection Using a Convolutional Neural Network
: 현재 ct사진에 쓰이는 cnn의 성능에 대한 글 (x-ray가 더이상 쓰이지 않는 이유)
x-ray와 비교하였을 때 ct 스캔에 쓰이는 cnn네트워크의 강점은 빠른 속도와 미묘한 골절까지도 찾아낸다는 사실, 하지만 여전히 남아있는 한계점은
" False negative, 즉 골절이 있는데 놓치는 경우가 많다는 것 "
골절을 놓치는 원인
1-1. 육안 골 전이의 영역들을 간과
1-2. 척추 앞 부종(prevertebral edema)과 디스크 확장(discspace widening)과 같은 2차 징후 때문에 골절이 잘못 식별되는 경우가 많다.
1-3. 선천적 이상도 골절로 잘못 식별되는 경우 많음
1-4. 골절 수술 이후 변화 (postsurgical defect)와 골절을 구분하지 못한다는 것
2. Current development and prospects of deep learning in spine image analysis: a literature review
- 현재까지 척추 이미지 분석에 쓰이는 네트워크 구조를 다룬 84개의 선행 연구를 분석한 논문에 따르면, 네트워크의 35.4%는 진단(분류), 30.4%는 segmentation, 22.8%는 detection이 차지하고 있었다. 그 중 classification의 경우 CNN과 U-Net, FCN, LSTM순서로 많이 쓰였으며, segmentation에서는 U-Net, CNN, LSTM, FCN순으로 많이 사용되었다.
- 척추 이미지 분할 및 분류에서는 CNN이 high-level 피처를 배우고, 압도적으로 좋은 성능으로 전반적인 정확도를 올려주었다.
3. Rib 골절 - nnU-Net & Densenet
- nnU-Net으로 segmentation을, DenseNet으로 classification을 진행한 연구
- segmentation으로 골절이 발생할 수 있는 부위인 갈비뼈 부분만 generate한 다음, 그 내에서 분류를 진행하여 정확도를 높였다.
- 갈비뼈 진단에 있어서도 CNN에 기반한 자동화 기술이 segmentation과정 없이도 99.1%의 정확도를 달성하고 있었다.
- CNN 이외에도 FracNet이라는 갈비뼈 진단을 위해 만들어진 네트워크가 존재하였는데, 이 네트워크는 3D U-Net에 기반하여 segmentation을 하고, ResNet을 분류 모델로 사용
- 하지만 기존에 있던 갈비뼈 골절 네트워크는 대부분 two-dimensional basis에서 시행되었다는 것이 단점, 본 연구에서 nnUnet과 DenseNet으로 구성된 알고리즘을 제안하게된 이유가 되었다.
- 모델에 대해 자세히 설명하자면,
- 첫번째 단계로 골절 nnU-Net을 Segmentation모델로 선정, 훈련하여 골절 후보 지역의 범위를 좁혔다. 즉, False Negative regions가 줄어든 좁혀진 범위 내에서의 데이터를 입력 이미지로 하여 후에 분류기의 분류 대상 부위를 줄여서, 최종적으로 갈비뼈 골절 인식의 정확도를 높일 수 있도록 하였다.
- ReLu를 leaky ReLu로, Batch Norm을 Instance Norm으로 대체하였으며, 첫번째 과정에서 random negative samples 수가 부족하였기 때문에, 2단계에서 골절 부위 FP를 제외시키는 과정을 거친 후, 마지막 3단계에서 모델을 평가하고 풀링 층의 개수, 입력 이미지의 해상도 등 파라미터를 설정하여 골절 분류 모델로 3D DenseNet을 사용하여 최종적으로 95%의 identification rate와 5%의 FP rate를 달성하였다.
- 2-stage rib fracture recognition model 2단계
- train to generate 골절 후보 부위
- deep learning segmentation model을 훈련시켜서 갈비뼈 골절 후보 region을 generate하는 부분
- train classification model
- 골절인지 아닌지 판단
- 첫번째 단계에서 뽑은 segmented local fracture regions를 분류하는 단계
- classification model을 훈련시키는 부분
- 결론⇒ 갈비뼈 골절 발견의 정확도를 높이고, FP와 FN을 줄여 골절 부위 발견에 있어서 의사들에게 도움
- train to generate 골절 후보 부위
Related Work
-
- ConvNets in a 2.5D manner
- 이미 ConvNets가 자동으로 posterior 골반 골절을 발견하는데 쓰이고 있음
- 위 방법은 identification의 sensitivity를 개선하는데 효과적
- DCNN(Deep CNN)
- 위 방법은 골절이 있는 환자들의 x-rays를 해석하는데 도움을 주고 misinterpretation 비율을 줄여주었다.
- CNN
- CNN에 기반해서 흉요추(thoracolumbar) 골절을 발견하는 자동화된 기술이 제시됨 ( segmentation없이도 정확도 99.1% 달성)
- ResNet & VGG
- 골절 부위 찾는데 SURF(Speeded up robust features) 알고리즘으로 ResNet을 더 잘 수행
- FracNet (갈비뼈 골절 발견을 위해 만들어진 네트워크)
- base는 classical segmentation network인 3D UNET
- which is improved by sampling strategy during trianing
- sensitivity 92.9%를 달성
- 시간 절약
- ResNet-based region proposal phase
- base는 classical segmentation network인 3D UNET
- Inception V3, ResNet50, MobileNetV2, VGG16
- 골절되지 않은 acute, aged한 ribs를 분류할 떄 쓰임
- Inception V3를 구성하는 7개의 블록이 정확하고 빨랐으며, 5-fold CV와 정확도는 96%, macrosensitivity는 94% 달성
- ⇒ (효과) 이러한 자동 갈비뼈 골절 시스템을 적용하여
- radiologists의 performance가 80.3%에서 91.1%로 증가함
- sensitivity는 62.4%에서 86.3%로 증가하는 등의 효과
- ⇒ (한계) 3D 정보를 잃고 2D basis에서 시행되었다는게 한계점
- ConvNets in a 2.5D manner
- (but most of the studies summarized above were conducted on a two-dimensional basis, losing three-dimensional information. Therefore, we propose an algorithm for automatic rib fracture recognition with nnU-Net and DenseNet in this study.)
따라서 여기서는 위의 선행연구의 한계를 극복할 수 있도록 nnU-Net과 DenseNet을 사용하였다고 함
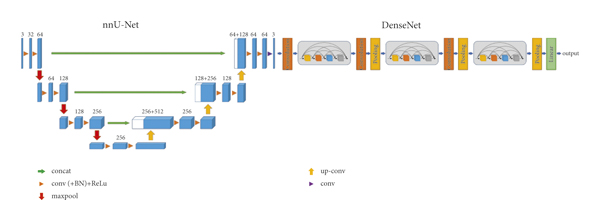
모델에 대한 설명
stage 1 : 갈비뼈 골절의 Regional segmentation
- 골절 후보 부위 선정 단계 (Fracture region)
- 3D CT 갈비뼈 골절 훈련 데이터셋
- nnU-Net을 분할 모델로 선정, 훈련시킴
- 훈련 이후 검증 데이터셋의 갈비뼈 이미지를 예측하여 골절 부위 후보 선정 ⇒ Determine segmented regions’s FP
a. 전처리
-
- 인스턴스 분할로 이미 라벨링 되어있는 데이터임 (우리도!..)
- 이미지가 블러처리되어 알아보기 힘들어 골절 발견이 더 어려웠기 떄문에, 5가지로 라벨링 되어있던 것을 2가지로 줄여서 갈비뼈 골절 분할의 정확도를 높임. ⇒ category가 아닌, binary fracture region segmentation (우리는 category segmentation)
- 이미지 해상도가 다 달라서 voxel X spacing 곱해서 size 새로 만듦
- 이미지들 표준정규화 시킴 Category label processing
b.손실 함수
-
-
- 다이스 계수 (Dice similarity Coefficient)로 분할의 효율성과 샘플 두 개에 overlap되는 정도를 측정
- 픽셀의 일부가 잘못 예측될경우, loss value와 gradient에 큰 영향을 미치기 때문에, 손실 함수를 다음과같이 변화시켜 개선
- 다이스 계수 (Dice similarity Coefficient)로 분할의 효율성과 샘플 두 개에 overlap되는 정도를 측정
-
기존의 다이스 계수

- Lce는 error loss arising from binary cross-entropy를 의미
- Lcontour은 골절의 3D profile의 error loss를 의미
변화시킨 다이스 계수

c. 모델 아키텍처
- nnU-Net이 experimental framework로서 쓰임
- nnU-Net은 robust adaptive framework (2d,3d unet기반)
- 이 프레임워크는
- 전처리(resampling, normalization)와
- 훈련 (손실, 옵티마이저 setting, 데이터 증강),
- 추론 (patch-based strategy, test-time-augmentation integration, model integration),
- post processing (enhanced single-connected domains)
- 에 모두 쓰임
- original Unet 구조를 변화시킨 것이지, 새로운 structure을 adopt하지는 않음(ex. 잔차 연결, …)
- 이 프레임워크는 유넷의 3가지 버전을 포함
- 2D Unet
- 3D Unet
- 3D cascade Unet
- 이 unet은 첫번째 단계에서는 down-sampled된 해상도가 낮은 이미지에 대한 coarse segmentation을 수행하고,
- 두번째 단계에서는 첫번째 단계를 합쳐서 fine-tuning에 사용함
- original Unet과 비교했을 때 여기서 사용한 Unet은 ReLu를 leaky ReLu로 대체하고, Batch Norm을 Instance Norm으로 대체한 모델이라는 점이 차이점
=> 사후 처리 Post Processing
- 훈련시킨 이후에 overlap regions in the sliding prediction 을 처리 - 여러번 예측하기
stage 2 : Fracture Area FP Exclusion
- 첫 단계에서 random negative samples가 부족했기 때문에, 2단계에서 FP만 제외시키는 네트워크가 필요함 (FP 비율을 줄이기 위해서)
- 따라서 2단계에서는 골절 부위의 FP를 제외시키고, 골절 예측에 있어 두번째 판단을 가능케 하는 단계임
stage 3 : 모델 평가 / 파라미터 setting
- 평가
- Dice 계수
- IoU (<=> mIOU[mean Intersection Union] )
- ASSD (Averate Surface distance) : 의료 이미지 분할에서 쓰인다고 함
- Hausdorff distance (HD) : 픽셀 하나하나 맞췄는지 여부보다는, 전반적인 추세를 맞췄는지 여부를 보기 위함 => 메트릭(거리) 공간의 두개의 부분 집합들 사이의 차이를 측정,점으로 이루어진 두 집합 간의 거리를 결정하는 방식
- 평균적으로 seg IoU가 0.99가 나오는게 중요한 것이 아니라, seg를 수행하며 한 사람의 폐암 환자도 놓쳐서는 안된다는 것이 당연히 더 중요함
- 0.99 mIoU나 0.98의 mIoU나 사람은 구별 못하지만, 놓치는 false negative case는 알 수 있다.
- 그래서 class-wise sensitivity, specificity도 함께 봐야한다.
- 특히 의료 영상 분석에 있어서는 false negative를 줄이는 것이 중요하기 때문에 여러가지 지표를 조합해야함
- 파라미터
- 데이터 전처리 이후에, pooling layers의 개수, input 이미지의 해상도, 그리고 2D Unet과 3D Unet의 평균 이미지 사이즈를 결정
선행 연구
***0. Current development and prospects of deep learning in spine image analysis: a literature review (79개 리뷰)
https://qims.amegroups.com/article/view/92987/html#figure9
Current development and prospects of deep learning in spine image analysis: a literature review
Current development and prospects of deep learning in spine image analysis: a literature review
qims.amegroups.com
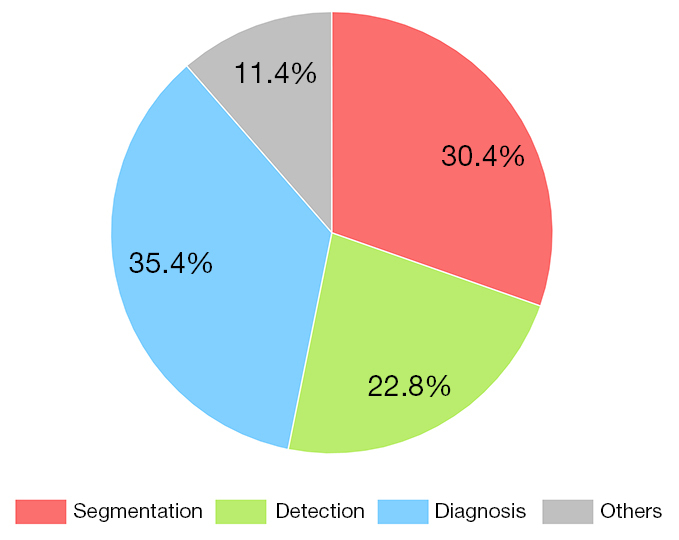

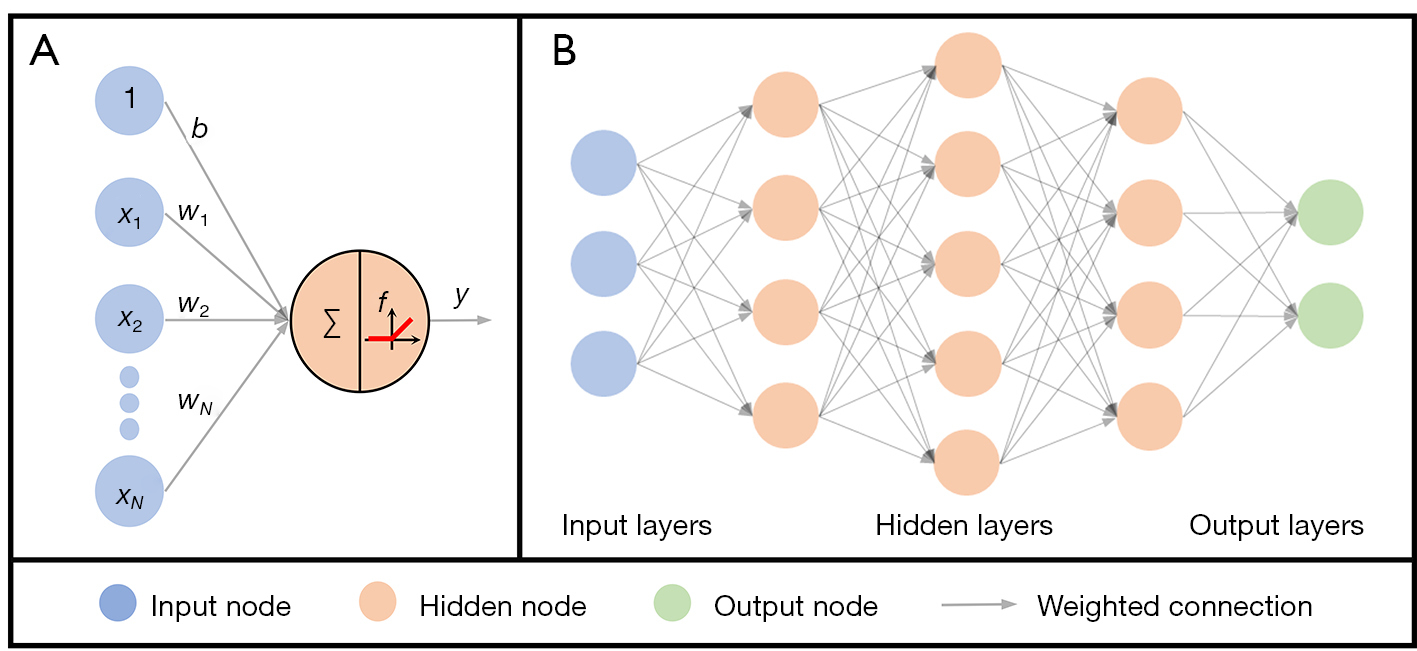
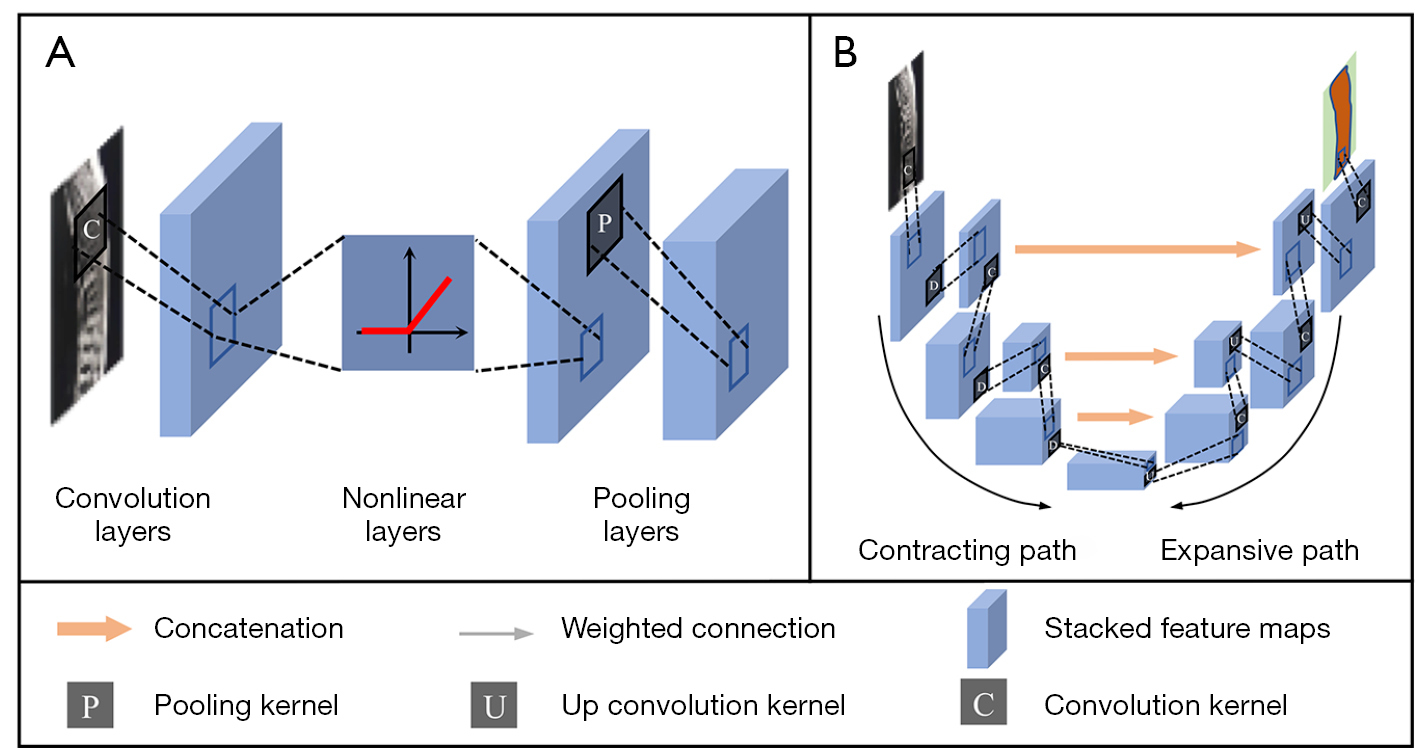
- DFNs are fundamentals for many DL models
- CNN, U-Net has a contracting path for image downsampling and an expansive path for image upsampling
- Convolutional neural network (CNN) is one of the most popular networks in spine image analysis. It consists of convolution layers, nonlinear layers, and pooling layers
- As the nonlinear layer is the same as DFNs, the following discussion focuses on the structures and functions of the other 2 layers.
- The U-Net is a typical CNN for image segmentation (42), which has a contracting path for image downsampling and an expansive path for image upsampling
- For spine segmented tasks, through the concatenation, multi-scale features are obtained to increase contrast and reduce blurred borders between vertebrae, IVDs, and the background, leading to more accurate spine segmentation

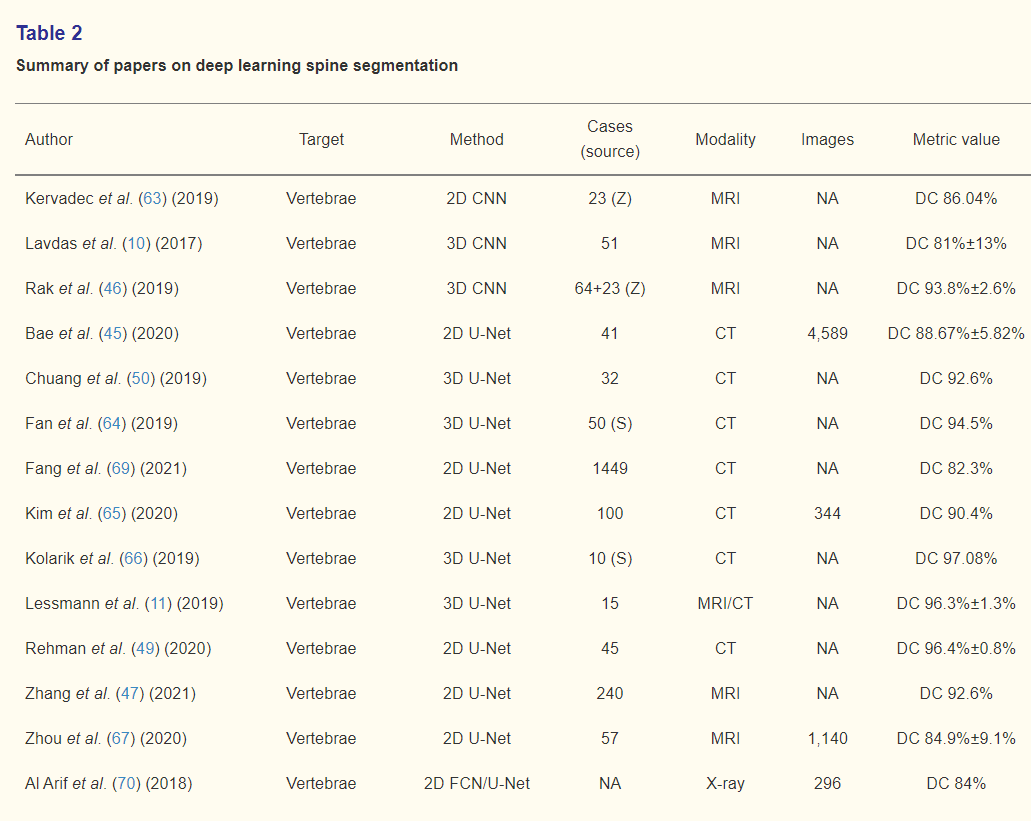
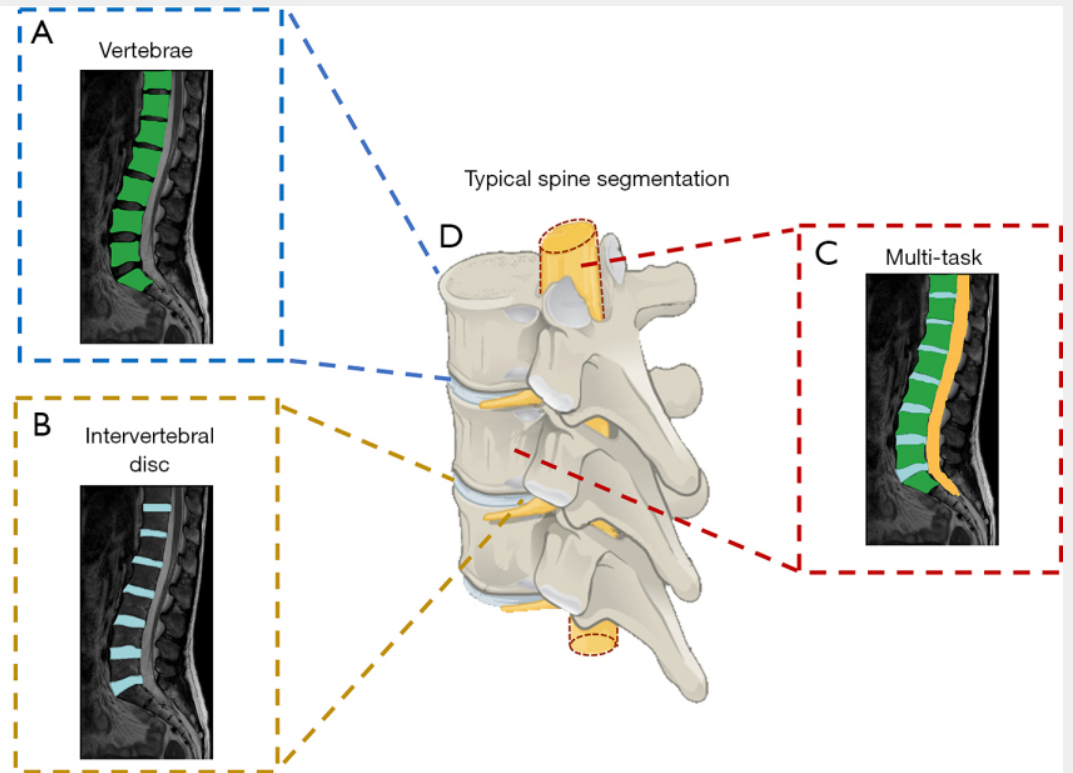
- fully convolutional network (FCN) and 2D U-Net to enable global localization, center localization, and vertebrae segmentation in a single thread.
- Tam et al. proposed the holistic multi-task regression method using multi-scale and fused image features and achieved outstanding portability and adaptability. To realize the segmentation and classification of multiple vertebral bodies simultaneously, Xia et al. designed a 3D FCN with coarse segmentation and a cascaded CNN with fine segmentation.
1. CT Cervical Spine Fracture Detection Using a Convolutional Neural Network
https://www.ncbi.nlm.nih.gov/pmc/articles/PMC8324280/
CT Cervical Spine Fracture Detection Using a Convolutional Neural Network
Multidetector CT has emerged as the standard of care imaging technique to evaluate cervical spine trauma. Our aim was to evaluate the performance of a convolutional neural network in the detection of cervical spine fractures on CT.We evaluated C-spine, ...
www.ncbi.nlm.nih.gov
2. Vertebral body insufficiency fractures: detection of vertebrae at risk on standard CT images using texture analysis and machine learning
https://link.springer.com/article/10.1007/s00330-018-5846-8#Bib1
3. An Algorithm for Automatic Rib Fracture Recognition Combined with nnU-Net and DenseNet
https://www.hindawi.com/journals/ecam/2022/5841451/
An Algorithm for Automatic Rib Fracture Recognition Combined with nnU-Net and DenseNet
Rib fracture is the most common thoracic clinical trauma. Most patients have multiple different types of rib fracture regions, so accurate and rapid identification of all trauma regions is crucial for the treatment of rib fracture patients. In this study,
www.hindawi.com
4. 경추 골절의 원인과 형태에 따른 위치와 빈도의 비교
https://scienceon.kisti.re.kr/commons/util/originalView.do?cn=JAKO199322138610893&oCn=JAKO199322138610893&dbt=JAKO&journal=NJOU00290714
원문보기 - ScienceON
scienceon.kisti.re.kr
5. Segmentation and Identification of Vertebrae in CT Scans Using CNN, k-Means Clustering and k-NN
6. Diagnostic Accuracy and Failure Mode Analysis of a Deep Learning Algorithm for the Detection of Cervical Spine Fractures
http://www.ajnr.org/content/early/2021/06/11/ajnr.A7179
Diagnostic Accuracy and Failure Mode Analysis of a Deep Learning Algorithm for the Detection of Cervical Spine Fractures
BACKGROUND AND PURPOSE: Artificial intelligence decision support systems are a rapidly growing class of tools to help manage ever-increasing imaging volumes. The aim of this study was to evaluate the performance of an artificial intelligence decision suppo
www.ajnr.org
Aidoc의 C-spine이라는 경추 골절 ct이미지 분석에 쓰이는 소프트웨어에 대한 글인듯 ..